What’s This About 🤔
Welcome to NFL Predictions, a series where we dive into what it takes to make NFL predictions. In this first part, Winner by Record, we’ll explore how the team seasonal record impacts the likelihood of winning games.
As the series unfolds, we’ll develop tools and eventually create a predictive model to answer the ultimate question: Who’s going to win? Let’s get started!
Disclaimers
First, I’m relatively new to American football, with just three years of watching under my belt. While I still have plenty to learn, this fresh perspective allows me to focus solely on the numbers, free from bias.
Second, while I mention betting, I discourage gambling for profit. A small bet for fun or tracking predictions is fine, but gambling to earn money is addictive and harmful.
Summary
In this article, we explored whether a team's season win and loss record can predict game outcomes. In the end, we built a very simple ML model that predicts winners with 80% accuracy, covering about 40 cases per year.
The Data
For this series, we'll use the play-by-play data provided by nflfastR (Big shoutout to nflverse). The dataset contains 372 columns of play-by-play data, spanning from 09/12/1999 to 12/02/2024, with a total of 26 seasons, 6,898 games, and 1,154,352 plays.
Below is a quick sneak peek at the data.
As you can see, there is a lot of information ranging from passing yards per play to tackles with assistance.
For this first part of the series, we will focus solely on how records impact the current game results. To simplify the dataset, we will narrow it down to Vegas information and the current season record.
Below you can see a more reduced table, that we will use:
Vegas Odds
When it comes to predictions, most ML models are built to compete against Vegas lines. But why fight it when we can embrace the wealth of information Vegas provides? Sure, there are tons of bookies offering different odds, and public betting probably skews the lines. But for this analysis, the spread line from Pro-Football-Reference should do just fine. It gives us a solid baseline for how accurate Vegas can be and sets the stage for some exciting comparisons.
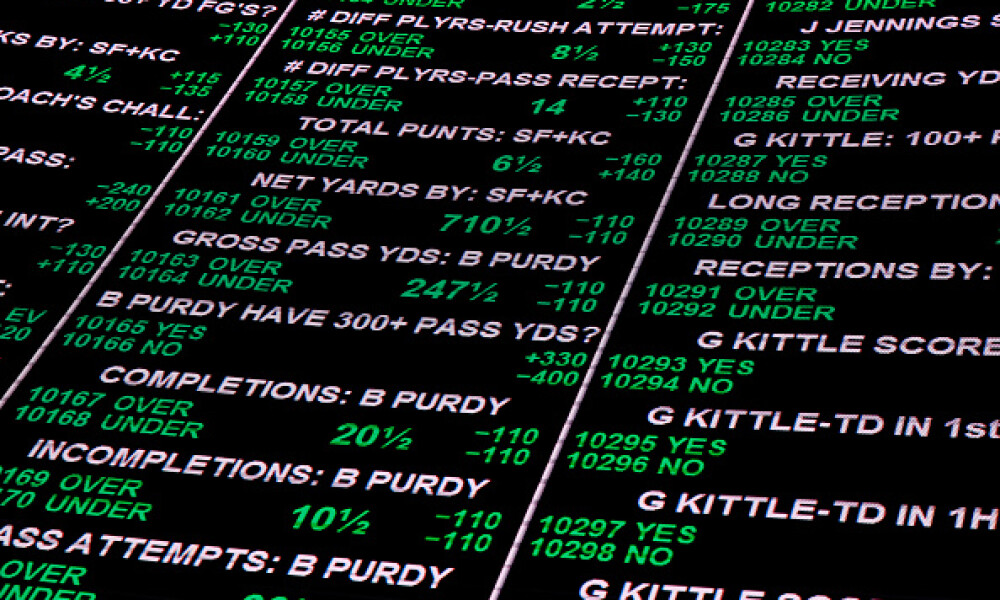
As shown in Fig. 1, Vegas' accuracy in predicting winners using the spread line was an impressive 66.3%.
Now, let’s take a look at how accurate Vegas is at projecting the winner based on the spread line. It’s important to note that in this part of the series, we are not focusing on the point spread itself. This means we’re not evaluating whether the spread was covered; we’re only predicting winners regardless of the points difference.
As expected in Fig.2, we can see that the larger the projected spread difference, the more accurate Vegas is at predicting the winner. However, over 50% of games have a spread line under 4 points, and for those, the accuracy is between 50-60%—not exactly impressive.
An interesting stat: in 94 instances where Vegas offered a spread of 15 or more, only 3 times did the underdog actually win. Those games were:
- Bills 27 vs 6 Vikings (September 23, 2018, Week 3)
- Dolphins 27 vs 24 Patriots (December 29, 2019, Wild Card Game)
- Jets 23 vs 20 Rams (December 20, 2020, Week 16)
Another game that sticks out—and one I remember all too well—was Week 14 of the 2023 season: my home team, the Miami Dolphins, faced the Tennessee Titans. Miami was riding high at 9-3, while the Titans were struggling at 4-8. Vegas gave Miami a 14-point spread, but we ended up losing 28-27. To make it worse, I had Tyreek Hill in my fantasy lineup, and he barely played in that game 😩.
The last time the @Titans played Miami on MNF, they mounted a 14-point comeback in the last 3 minutes of the game 😳
— NFL (@NFL) September 30, 2024
📺: #TENvsMIA – Tonight 7:30pm ET on ESPN
📱: Stream on #NFLPlus pic.twitter.com/rBKrieWrzN
The Record
Let’s dive into the goal of this article and find out whether a team’s record influences their chances of winning.
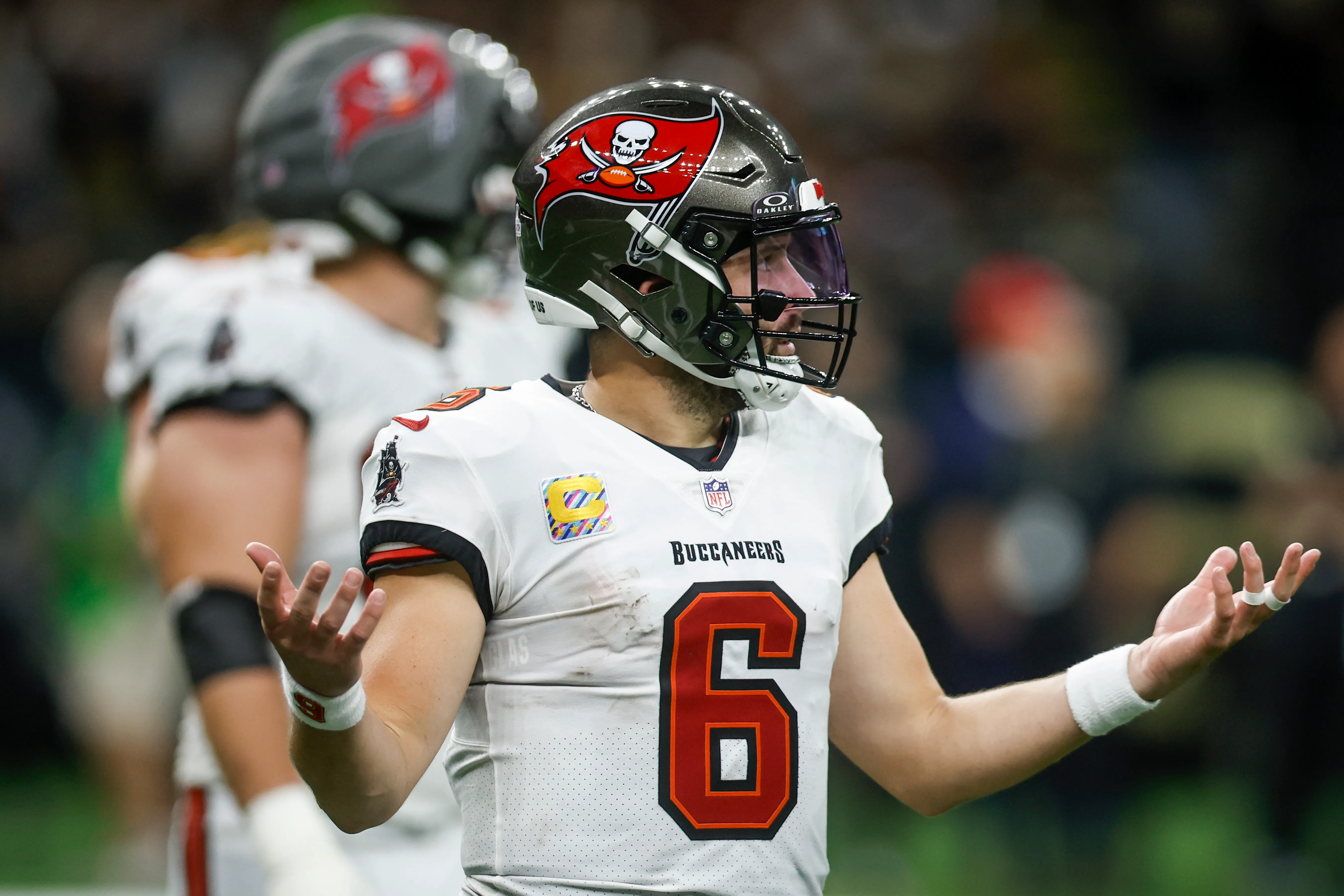
First, let's calculate the Pearson correlation coefficient of winning and winning margin against:
- Total Games (
record_total_games
) - Season Games Won (
season_winning_record
) - Season Games Lost (
season_losing_record
) - Season Winning Ratio (
season_winning_ratio
) - Opponent Season Games Won (
opponent_season_winning_record
) - Opponent Season Games Lost (
opponent_season_losing_record
) - Opponent Season Winning Ratio (
opponent_season_winning_ratio
) - Winning Ratio Difference (Season Winning Ratio - Opponent Season Winning Ratio) (
winning_ratio_diff
) - Whether the team is playing at home or not (
is_home
)
We'll also include the Vegas Spread Line for comparison.
As expected, Vegas knows what it's doing—the Vegas spread line is far more correlated to winning and winning margin than the winning games ratio. Additionally, winning ratios are more significant than the record itself.
But what about winning streaks? Do they have an impact in the NFL?
As shown in the table above, the opponent’s winning ratio has minimal correlation with the likelihood of winning. However, longer streaks—such as 12, 13, or 14 games—seem to have a more significant influence on determining the winner.
In other words, having a winning or losing record doesn’t directly impact the outcome in a noticeable way. But let’s dig deeper into the data to see if we can uncover any hidden patterns.
Now we’re getting somewhere! As we can see, when the winning ratio difference is small, anything can happen. But as we move toward the extremes—where (>0.25) a great team faces one that’s struggling—we start to see a clear trend emerge.
One interesting observation is that when the diff=1
, the pattern shifts. After inspecting the data, this happens early in the season when there are many unbeaten or winless teams. So, let’s try filtering the data to include only games after Week 3.
Well, this is something. Let's check correlations after Week 3 and when the winning ratio difference is greater than 0.25 or less than -0.25.
Now our data aligns closely with the Vegas spread, which is motivating enough to try building a simple ML model. And yes, we’ve significantly reduced our dataset—from 6,898 games to just 2,254 games—but hey, you can’t always win, right? 😅
Machine Learning
Now let's try to make a simple ML Model, we will use a Decision Tree Algorithm mainly because it is simple and very illustrative, and also we have a very small dataset with lower than 10000 datapoints.
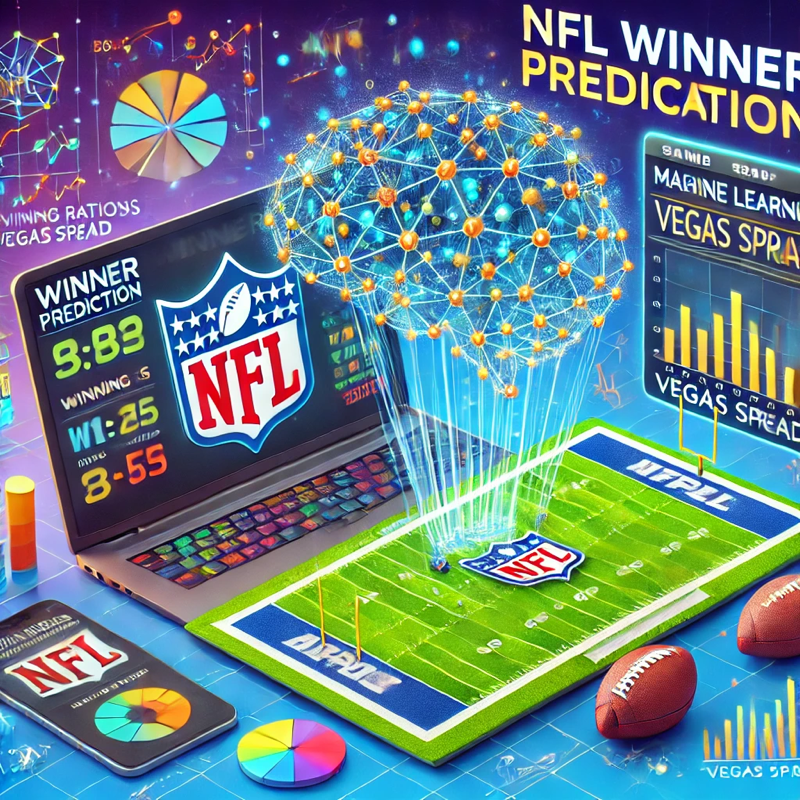
Decision trees are a type of supervised machine learning algorithm used for both classification and regression tasks. They work by recursively splitting the data into subsets based on the features that provide the most significant separation according to a chosen metric. This method is interpretable, as we can visualize how the tree splits the data and the decision-making process it follows. However, Decision Trees can be prone to overfitting, especially with complex datasets, so we’ll use techniques like limiting tree depth to ensure better generalization.
First, we will split the data. We will use data from 1999 to 2022 to train our Decision Tree, and then test performance with 2023 and 2024 data.
Fig. 5 shows the decision tree. We optimized the parameters using GridSearchCV, which means we tested several tree configurations to find the one that performed best. As shown, the tree has 3 depth levels. It first checks whether winning_ratio_diff > -0.029
, then evaluates if winning_ratio_diff > 0.216
, and finally considers whether the team is playing at home.
From 1999 to 2022, teams that were local and had a winning_ratio_diff
of 0.216 played 990 games and won 763 of them, achieving an impressive win rate of almost 80%.
Now, let's test how this simple rule would have done in 2023 and 2024.
As we see above, out of 64 instances where this condition was met, the 80% win ratio was maintained. In 51 of those cases, the winning team celebrated at home 🎉🎉🎉🎉.
Now, let’s move on and display the table of predictions.
Actually, in Week 13 of 2024, we could have hit a 7-leg parlay 😂.
Conclusions & Credits
We’ve concluded the first part of NFL Predictions. We achieved a solid result with a simple decision tree providing an 80% success rate in cases where it applied—around 35-45 cases per year. In the next part, we’ll dive into Points, Spreads, and Totals.